In the realm of video analytics, TraceVision is adopting a unique approach in hybrid edge/cloud computing. This sophisticated method synergizes the capabilities of on-device and cloud-based processing, offering a more efficient and scalable solution for real-time video analysis.
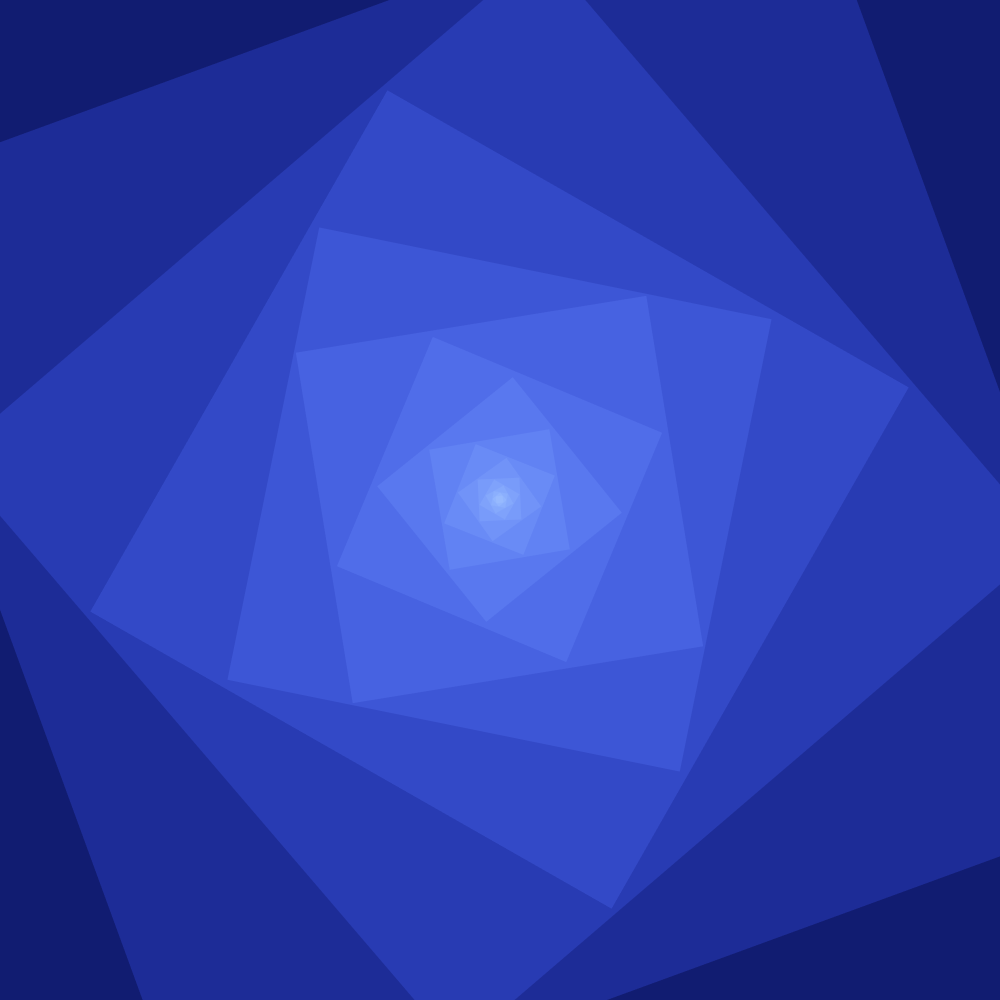
Introducing the Hybrid Edge-Cloud Ecosystem
Our latest innovation is a robust ecosystem that merges the localized computational prowess of edge devices with the vast analytical capabilities of the cloud. This synergy is not just a technological leap—it's a new paradigm in AI vision, offering businesses an unprecedented level of flexibility, scalability, and efficiency.
TraceVision's hybrid model employs a dual-processing strategy. On the edge side, AI algorithms run on users' phones, handling tasks optimized for mobile processing. This means tasks that require immediate response or are sensitive to latency are processed locally on the device. On the cloud side, more complex, computationally intensive tasks are offloaded to powerful cloud servers. This strategic division of labor between edge and cloud computing optimizes both speed and processing power.
A Technical Exploration of the Hybrid Model
The heart of this approach lies in its real-time processing capabilities. Mobile optimizations enable phones to process AI models rapidly, identifying and forwarding potential event highlights to the cloud server. This two-tiered process ensures that while the edge devices handle immediate processing, the server conducts in-depth analyses, processes heavy workloads, and distributes the results back to users' phones.
- Edge Devices: Embedded with Docker containers, these devices are the workhorses of on-site data processing, capable of executing AI algorithms with remarkable speed and efficiency.
- Edge Server/Gateway: Leveraging the orchestration power of Kubernetes, our edge servers act as the neural nexus, coordinating data flow, refining analytics, and maintaining seamless communication between the edge and the cloud.
- Network Edge: This layer represents the expanded reach of our computing capabilities, facilitating a more comprehensive and connected AI vision network.
- Hybrid Cloud: Culminating in the hybrid cloud, our infrastructure leverages Kubernetes to perform sophisticated data processing, enabling complex AI tasks and model training across a secure, scalable cloud environment.
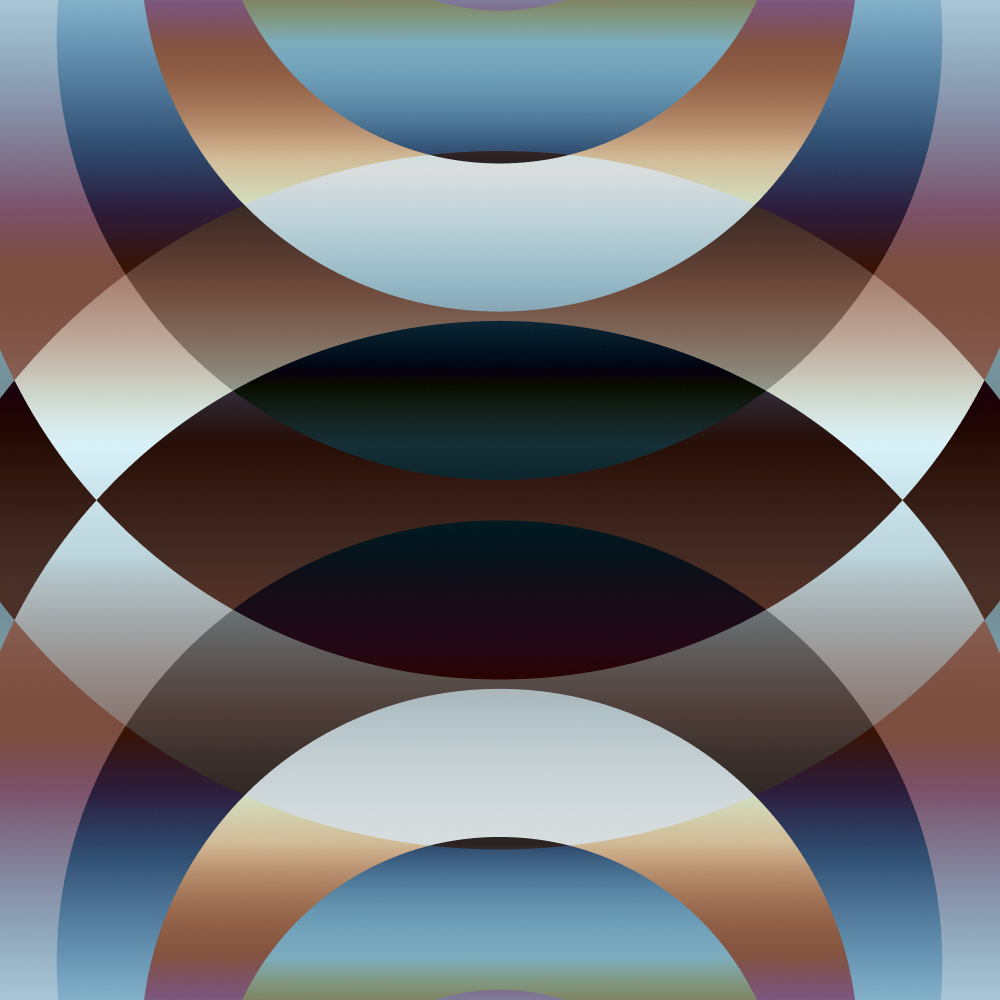
Operational Mechanics: A Closer Look
Consider a scenario where multiple phones are used to film a sports event. Each phone, running a dedicated app, performs quick on-device processing tasks like detecting key moments in the game. These devices are interconnected and communicate with a central server via WebSockets. The server, equipped with a robust web application, receives these initial findings, performs enhanced processing (like synthesizing multiple angles or applying advanced analytics), and then dispatches the polished highlights back to the phones.
Practical Applications: A Broad Spectrum
This hybrid computing approach finds its utility in diverse fields:
- Sports Analytics: It enables capturing and analyzing key moments from multiple angles, delivering near-instantaneous highlights to remote viewers.
- Security and Surveillance: Useful in identifying and reacting to security incidents in real-time, enhancing public safety.
- Event Management and Retail: Offers insights into crowd dynamics and behavior, aiding in better event organization and retail management.
The Future of Hybrid Computing in Video Analytics
TraceVision is committed to refining and expanding the capabilities of its hybrid edge/cloud computing model. Our aim is to make real-time video analytics more robust, efficient, and accessible across different sectors.